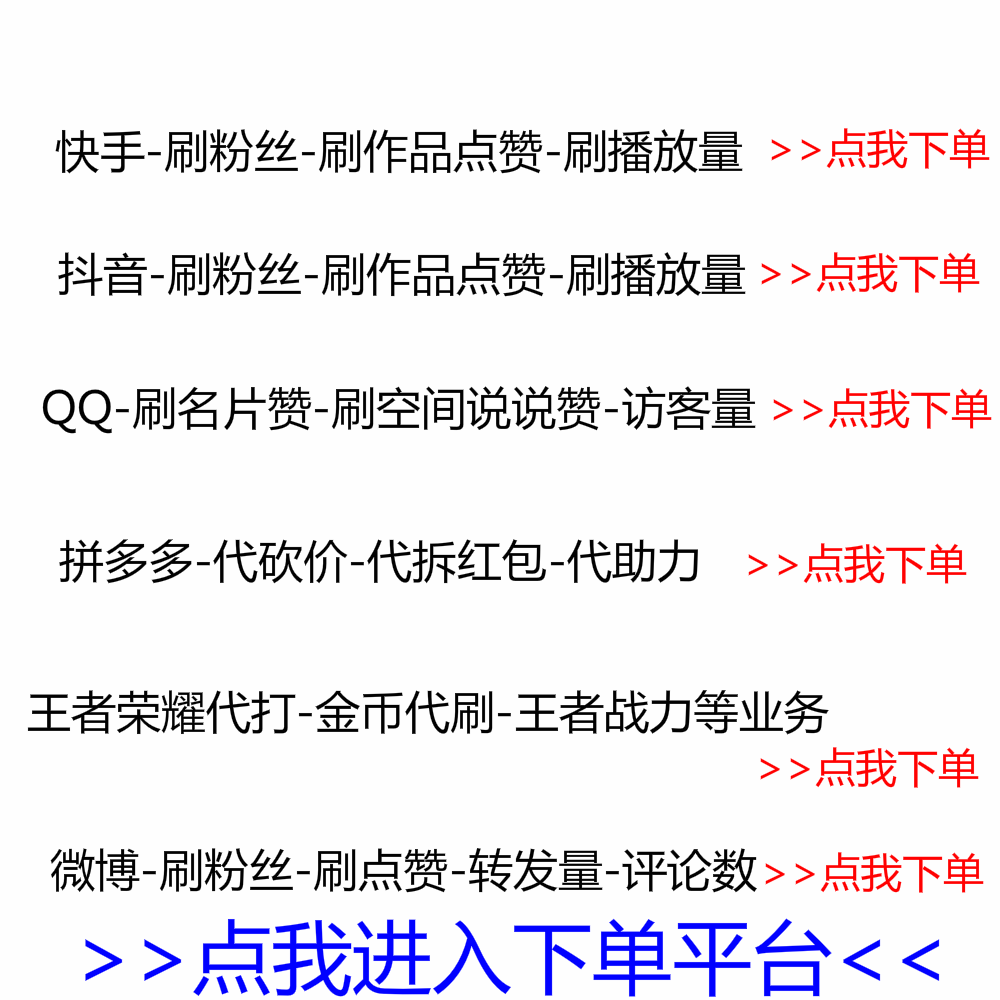
我之前上过演绎逻辑课,同意一共上三课。第二次教归纳逻辑,但是因为太懒,没有继续联系归纳逻辑。今天我会做的。这弥补了它。关于归纳逻辑,我主要向同学们介绍以下几个问题:第一,什么是归纳逻辑;第二,归纳逻辑的历史演变;第三,科学假设与归纳。
一、什么是归纳逻辑?
在我们之前关于演绎逻辑的讨论中,我们了解到演绎逻辑主要是说,在论证过程中,如果给定的前提为真,那么由演绎推理决定什么。得到的结论也是正确的,也就是说,演绎逻辑的主要功能不是保真,而是“传真”。
给定前提的真值从何而来?在这个问题的讨论中,你可以回到我之前讨论的关于真理概念的问题。我不会在这里详细介绍。从源头上讲,我们的知识应该说是来自于我们对世界的接触、观察和理解。实验积累的感官经验资料,以及在这些资料的基础上做出的概括。
为什么这么说?所谓知识,其实就是对某一类事物的属性或关系的完整描述。简单地说,这是一个普遍的真理。那么我们已经知道扣款的功能就是“传真”了。既然是“传真”,就必须有“真”才能传出去,“传”前的“真”需要靠归纳。它是通过直观的推测或对许多个体事物的观察进行的概括,本质上是归纳。
让我们以那个经典的三段论为例:
所有人都死了;
苏格拉底是个男人;
苏格拉底 底层会死。
这是一个经典的演绎推理。大前提:所有人都会死。这句话属于关于“人”范畴属性的一般真理。这个真理是如何得到的?通过归纳得到,其过程大致如下:
在我们过去的观察中,我们发现:
老子会死
孔子会死
故事会消亡
柏拉图会死
秦始皇将死
…………
并没有找到反例,所以我们进行全名判断:
所有人都会死。
之前的经典三段论之所以行之有效,是因为苏格拉底已经包含在每个人当中了。换句话说,在我们最初的归纳中,我们实际上已经考虑了苏格拉底的死亡。如果你进去,你会得到每个人都会死的真相。
所以我们可以清楚地看到,演绎的大前提来自于归纳(当然不是演绎推理的每个大前提都来自于归纳,但从根本上说,它必须来自于归纳),这是归纳逻辑的核心。
在这里,我们尝试对归纳逻辑和演绎逻辑进行以下三个层次的区分:
首先,从思维的角度来说,演绎是从一般推论到个别判断,或者从一般判断到另一个一般判断,例如:
1.所有的花都需要阳光,牵牛花也是一朵花,所以,牵牛花需要阳光;
2.如果所有的讨论都是关于政治的,那么所有的讨论都是意识形态的斗争,所有的讨论都是关于政治的,所以所有的讨论都是意识形态的斗争。
归纳是从个人判断推到一般判断,或者从个人判断推到另一个个人判断,例如:
3.老子会死,孔子会死,泰勒斯会死,柏拉图会死,秦始皇会死,…………所以,每个人都会死;
4.从第一天我认出,从天上,太阳从东方升起,第二天,太阳从东方升起,第三天,太阳从东方升起……直到今天,太阳从东方升起。所以,明天太阳会在东方升起。
其次,从前提和结论的关系来看,演绎推理是一种缩小推理,而归纳逻辑是一种放大推理。例如,我们看前面的例子1。牵牛花已经包含在所有的花中。既然都说所有的花都需要阳光,那么牵牛花这种花当然也需要阳光。让我们再看一下示例 3。最终结论涵盖了所有人的判断,大于所有前提给出的范围,也大于所有前提的合取范围。
那么我们要问,演绎和归纳哪一个可以提供新的知识?这需要从两个方面来讨论。首先是逻辑中的“新知识”。既然演绎是缩小推理,归纳是放大推理,演绎不能提供新知识,而归纳可以提供新知识;二、心理学 什么是心理学新知识?它指的是我们不知道或应该知道但不知道的知识。比如一个命题A需要经过一个很长的演绎逻辑链才能得到命题B,但是因为我们之前不知道这个逻辑链,所以命题B也是新的。知识,在数学上通过计算得到新的推理和证明,大部分属于后者,在这个意义上,演绎逻辑可以提供新的知识。当然,归纳可以提供新知识,无论是逻辑上还是心理上。
但是有同学会问,貌似归纳逻辑好像很厉害,但是为什么总觉得不靠谱呢?这将我们带到了第三层讨论。
第三,从推理的性质来看,演绎推理属于必然性推理,前提的真实性可以保证结论的真实性,而归纳推理属于概率性推理,前者的真实性不能保证结论的真实性。结论,前提只能在一定程度上支持结论。回到前面的例子,比如例子2,由于演绎推理是一种狭义推理,结论已经包含在前提中,演绎推理只是对结论进行“分析”,所以演绎推理是不可避免的。让我们再看一下示例 4。结论是关于明天的太阳。前提是无论收集多少关于太阳的数据,都无法覆盖明天太阳的数据。因此,我们永远不能说明天的太阳必须符合归纳推理。结论。
虽然归纳推理和演绎推理是有区别的,但我们应该清楚地看到两者是相辅相成的。演绎推理的一般知识的主要前提必须通过归纳推理从具体的经验知识中概括出来,在这个意义上,没有归纳推理就没有演绎推理。归纳推理也离不开演绎推理。归纳活动的目的、任务和方向不能由归纳过程本身来解决和提供。只有借助理论思维和人们先前积累的一般理论知识的指导,这本身就是一种演绎活动。此外,仅凭归纳推理不能证明必要性。在归纳推理过程中,人们往往需要运用演绎推理来证明某些归纳前提或结论。从这个意义上说,没有演绎推理就没有归纳推理。因此,正如恩格斯所指出的:“归纳与演绎,如同分析与综合,必然是相互关联的。不应该为了另一个而牺牲一个,而应该在该用的地方各用其用。而要做到这一点,只有注意他们的相互联系,他们的互补性。”
二、归纳逻辑的历史演变
从时间序列看,归纳逻辑传统(经典)归纳逻辑和现代归纳逻辑,传统归纳逻辑,起源于弗朗西斯·培根,终结于密尔;现代归纳逻辑由凯恩斯创立,由赖兴巴赫、卡尔纳普、科恩等人发展,利用概率论和公理化方法讨论归纳逻辑问题,现代归纳逻辑也称为概率归纳逻辑。
(一)亚里士多德的归纳法
如前所述,传统归纳逻辑的正式确立始于培根,而在此之前的古希腊、罗马时期和中世纪的归纳逻辑属于归纳逻辑历史上的萌芽期和萌芽期。归纳逻辑的发展。由于当时的相关研究比较分散和松散,所以在这一时期的研究中,主要是通过一些代表的观点来总结这一点。这一时期的一些成就。
作为屹立于古希腊文明中的哲学巨擘,完成了希腊古典哲学的大老板,亚里士多德不仅是形式逻辑的奠基人,也是归纳法的奠基人(请注意,我们说的是归纳法,不是系统的归纳逻辑),在亚当、德谟克利特、苏格拉底、柏拉图之前都提出过一些归纳的思想,但并不完整和系统,如苏格拉底归纳法的底层只是对归纳法的定义伦理学概念,以及证明或反驳有关道德规范的一般命题的方法。亚里士多德的归纳法大致有以下几种,即完全归纳法、简单列举归纳法、直觉归纳法、实例归纳法。让我们做一个概述:
(1)完全归纳(归纳的三段论)。严格来说,完全归纳因为前提的真实而可以保证结论的真实,是必然性推理,应该属于演绎推理的范畴,但我们还是可以在这里介绍一下。
亚里士多德在《前分析》第 2 卷第 23 章中说:“归纳法,或从归纳法派生的三段论,它是利用三段论借用另一端与中间建立关系。 . 例如,如果 B 是 A 和 C 之间的中间,它在于证明 A 通过 C 属于 B。这就是我们进行归纳的方式。假设,假设 A 代表长寿,B 代表无胆,和 C 为个体长寿动物,如人、马、骡子。则 A 属于所有 C,但 B(不含胆汁)也属于所有 C。如果 C 和 B 可以调换,则扩展中间不大于,则 A 必然属于 B。因为已经证明,如果两个谓词属于同一个主语,并且结尾与其中一个转置相同,则其他谓词属于转置谓词。我们必须将 C 理解为由所有特殊事务组成,因为归纳是通过枚举所有情况进行的。”这是第一个有直接前提的三段论的成立:因为凡有中间词的地方,三段论都由中间词进行;当没有中间词时,通过归纳。在某一方面,归纳法与三段论相反:因为后者用中间词来证明大词属于第三项,而前者用第三项来证明大词属于中间词。在自然顺序中,中间词的三段论是最先熟悉的,而归纳法的三段论对我们来说更清楚。”
有兴趣的同学可以自行分析。由于时间问题,我不想扩展它。说了这么多,我举个简单的例子,你就明白了。例如,太阳系中有九颗行星。我们调查九颗行星并得到:
水星沿椭圆轨道绕太阳公转;
金星绕太阳公转;
地球在椭圆轨道上绕太阳公转;
火星在椭圆轨道上绕太阳公转;
土星是它在椭圆轨道上绕太阳运行;
木星在椭圆轨道上绕太阳公转;
天王星在椭圆轨道上绕太阳公转;
海王星在椭圆轨道上绕太阳公转;
冥王星在椭圆轨道上绕太阳运行;
因为太阳系一共有九颗行星,所以我们说:
太阳系中的所有大型行星都以椭圆轨道围绕太阳运行。符号形式化为:
S 1 ——P
S 2 ——P
…
S n ——P
(S 1 S 2 ……S n 都是 S 类分子)
所以,S—P。
由于我们已经彻底调查了该类别中的所有对象,因此得出的一般判断是不可避免的。说白了,这样笼统的判断,其实是一种孤独,也就是一派胡言。因为所有的对象都被检查过了,把它们包含在一个句子中就等于说一个重言式。当然,也正是因为如此,推理本身是不可避免的。
(2)简单枚举。亚里士多德说:“我们要分清论据有多少种。一方面有归纳,另一方面有推理。我们已经清楚解释了什么是推理之前。归纳是一个从一般到一般的过程。
简单枚举也称为不完全归纳。适用场景比较窄,只适合研究有限集,而对于无限集,完全归纳法显然是无能为力的。简单的枚举方法也很简单。我们一开始说的是简单的枚举法,直接象征Just:
S 1 ——P
S 2 ——P
S 3 ——P
……
p>
S n ——P
(S 1 S 2 S 3 ……S n 是类S的部分对象,枚举中没有遇到相反的情况。)
所以,S—P。
(3)直觉归纳法。亚里士多德说:“我们必须通过归纳法获得初始前提;因为感性知觉注入普遍的方法是归纳法。现在,我们掌握真理的心理状态事物中,有些永远是真的,有些可能是假的——比如意见和计算,而科学知识和直觉总是正确的;此外,除了直觉之外,没有其他思想比科学知识更确定,初始前提更比证明可知,所有的科学知识都是推论的。从这些考虑可以得出结论,不可能有任何关于前提的科学知识,并且由于只有直觉比科学知识更真实,所以理解初始前提——结论的将是直觉:证明不能是证明的原始来源,因此不能是科学知识的科学知识因此,如果直觉是唯一一种真正的思想除了科学知识,它是科学知识的原始来源。而科学的原始来源拥有原始的基本前提。科学作为一个整体,与所有事实的原始来源相同。简单来说,所谓直观归纳,就是我们对被观察对象的直接而透彻的理解,当然也是基于真实经验的,但它不像完全归纳或不完备。归纳法可以一一考证,但大脑的灵感突然冒出来,直接提出了一个“假设”。直观归纳法可以理解为科学方法中假设的前兆,后面我们会详细讲。严格来说,这应该是我们的大脑在对我们感知到的杂乱无章的数据资料进行自动排序后获得的共同意识。本质上也是感应的产物,只是这种感应不是通过理性的外在观察,而是大脑的速度。处理。
(4)例子。亚里士多德说:“如果用第三个词这样的词来证明大词属于中间词,我们就有一个‘例子证明’。这需要双方都知道中项属于第三项,第一项属于与第三项类似的项。例如,假设A代表邪恶,B代表对邻国的战争,C代表雅典对底比斯,D代表底比斯反对福奇。如果我们要证明与底比斯作战是不好的,而与底比斯作战就是与邻居作战,那么显然与底比斯作战是不好的。显然,B 属于 C 和 D(因为在这两种情况下,战争都是针对邻居的),而 A 属于 D(因为与福奇斯的战争的结果对底比斯不利)。但是 D 证明了 A 属于 B。如果关于中间词与结尾词的关系的信念应该由几个相同的实例产生。因此很明显,当两个特定的事物是附加到同一个术语,并且其中一个是已知的,通过例子的论证既不是部分对整体也不是整体部分,而是部分对部分的推理。它不同于归纳法,因为归纳法是从所有特例出发,证明大词属于中间词,而不是将三段论的结论应用于小词。并且实例论证不是这样使用的,它的证明也不是从所有特殊情况中得出的。”
这还是老规矩,我对自己的分析感兴趣,我会简化时间问题。示例方法与类比参数类似,符号为:
A有属性a、b、c、d
B有属性a,b,c
所以,B 有属性 d。
从亚里士多德到中世纪,归纳法的成就并不多。古希腊哲学家伊壁鸠鲁及其学派提出了一些归纳理论,主要有观察、类比和假设方法的理论,这里不再赘述,我们直接讨论培根。
(二)弗朗西斯·培根的排他性归纳法
关于培根的感应法,这里我们需要做一个简单的背景调查,以明确培根的感应法不仅仅是石缝中的。 @1)培根的归纳自然科学基础
在现代欧洲,随着资本主义的兴起,生产能力得到了极大的发展。在此基础上发展了现代自然科学。
1543年,哥白尼发表了震惊世界的《天体运动学说》,提出了“日心说”,开始动摇了基督教宇宙学的基础,自然科学逐渐脱离神学;第谷; 1577年,他观测到一颗巨大的彗星,证明它比月球还远,说明天界并不完美。他的观测资料直接影响了最先进星表的出现;第谷的助手开普勒接手第谷的 1609 年和 1619 年,他发现了行星绕太阳运行的椭圆轨道定律,并提出了著名的开普勒三定律,发展了哥白尼的日心说,确立了太阳系的概念,并利用椭圆轨道轨道取代了完美的圆形轨道; 1610年,伽利略用自制望远镜观测天体,发现了许多新的天文现象,对支持哥白尼学说具有重要意义。伽利略在物理学上也取得了许多成就,如落体运动定律、摆等时性、抛物线运动等;伊丽莎白女王的医生吉尔伯特用实验方法发现了磁倾角,他还提出了质量和力等新概念;比利时维萨利乌斯 1543年,他发表了《论人体结构》,这是现代史上第一部人体解剖学著作;英国医生哈维提出了“循环”的概念,他发现血液循环不依赖于推测,而是依赖于解剖学方法的运用。心的观察。
这些是弗朗西斯·培根建立归纳法时自然科学领域的一些主要成就。 Gilbert 的工作在 The New Tool 中有所提及,他也曾与 Harvey 的患者一起工作,因此可以合理地断言,基于观察和实验的综合新方法应该对 Bacon 的归纳有积极的启发。
(2)培根的归纳思想
1620年出版了未完成的作品《大复兴》,而《新工具》是培根作品的六个部分,第二部分是本书已完成部分的主体。
p>
新文书不同于亚里士多德的“文书”,提出了亚里士多德的三段论,质疑和否定的目的是为人类理性开辟一条与以往完全不同的道路,使人类的思想能够对自然行使与生俱来的权威的东西。
对“新工具”的批判 他认为三段论的演绎方法不能帮助人们寻找真理;它只求在辩论中赢得对手,而不是在行动中征服自然。为此,培根在书中全面详细地提出和阐述。他介绍了他的新的逻辑方法,即归纳法,并将他关于这种方法的论述称为“新工具”,以表明它不同于亚里士多德的《论仪器》,后者主要基于演绎逻辑。
他强调,要想得到真相,就要通过观察和实验的方式,搜集大量的资料,然后运用精髓和表、差表、度的“三表法”表整理所获得的感性材料,即通过分析、比较、排除,排除非本质的事物,最终获得对本质的理解。同时,归纳和泛化必须一步一步来实现,不能任由理性跳跃。
培根的归纳法分为三步:
第一步是通过观察和实验,尽可能全面地收集对事物的感官体验。他说:“首先,我们必须准备一部自然史和实验史,才能完全更好。这是一切的基础;因为我们不是去想象或假设,而是去发现自然在做什么或我们能做什么。告诉它去做。”
第二步,把收集到的材料进行整理整理。如何排序?培根提出了著名的“整理、分析、比较材料的方法”,也称为“寻找事物因果关系的方法”,即“三表法”。
表 1:“本质和有表”。列出具有一些共同属性 A 的示例;
两个表,“差异表”。其他相似之处,但不具备上表所考察的相似性质A;
第三步是使用排除法。这一步分为三个小步骤:
第一步是消除那些不重要的属性。排除了哪些属性?
p>
1.“有表”中不存在的属性;
2.“差异表”中存在的属性;
3.属性A减少但增加;
4.属性 A 增加但减少。
第二个小步骤,通过九个辅助辅助方法重新制定上述排除步骤;
第三小步,排除了应该排除的属性后,积极提出共同属性A。
(3)培根感应的评价
历史:培根的归纳法奠定了经典归纳逻辑的基础,对后来经典归纳法的发展做出了巨大贡献。没有。
培根的归纳逻辑思想具有以下三个基本特征:
首先,在观察和实验的基础上,首次将逻辑与科学方法相结合,将归纳法与观察、分析、实验紧密结合,寻求因果联系,归纳法不再是简单的枚举法,而是一种科学归纳法。
二、实事求是 对通过观察和实验收集的材料进行整理、分析、比较,强调认知过程及其结果必须建立在可靠的基础上。
第三,通过科学归纳,认识事物的起因——认识自然规律。
局限:归纳讨论不够详细,缺乏严谨的定义,没有形成明确的归纳推理形式和规则;对自然的讨论不足,为休谟提出归纳困难留下了后门;他不承认归纳与演绎之间的联系,忽视和贬低演绎的作用;只注重定性分析,缺乏定量分析,缺乏对数学方法的理解。分析和关注度不够等等。
然而,培根在归纳逻辑方面的开创性成就和他对逻辑科学发展的杰出贡献,将与亚里士多德的演绎逻辑一样广为人知。在世界上。
(三)米尔斯5定律
培根之后,英国天文学家约翰赫歇尔举了许多自然科学的例子,证实培根的归纳法对培根的方法起到了很大的宣传作用;英国科学史学家威廉·休厄尔在 1840 年出版了《归纳科学的哲学》一书。培根的归纳法做到了公正。在综合评价中,他认为科学探索不是一个简单的经验概括的过程,而是一个分析实验事实、提出假设、验证假设推论的过程。
在培根和赫歇尔的基础上,密尔在休厄尔等人的归纳法的基础上,提出了寻找因果关系的归纳法。 “五法求因果”遵循一个原则,即“因果普适性”。也就是说,任何现象都必须有它的原因,也必须有它产生的结果;现象是遗传的,原因在前,结果在后。在这个总原则下,他提出了以下五种方法,即:从众法、差异法、从众法和差法、余法法。协变法。以下是五种求因果的解释。
(1)合约方法
如图所示,对于现象“*”,列举1-5个场合,fit方法从必要条件的意义上判断原因,我们先排除非发生的条件表中现象“*”,若3次都没有A,则排除,同理排除B、C、D、E、G从一般到特殊的逻辑方法,剩下的F为该现象的必要条件“ *”,则条件 F 是“*”现象的可能原因。
注意事项:
1.每个场合除了F还有条件吗?
2.检查的场合越多,结论就越可靠。
(2)差分法
如图,对于现象“*”,列举1-2次,区别法是在充分条件意义上判断原因,即有条件xx时从一般到特殊的逻辑方法,必有一个现象“*”,通过场合,可以排除A、B、C、D、E、F。只有剩下的 G 是“*”现象的充分条件,所以 G 是“*”现象的可能原因。
注意事项:
1.这两种情况是否还有其他条件也是充分的;
2.注意单因是不是复合因。如果是复合原因,其中的分子原因也会影响现象的出现。
( 3)适合差异使用
如图,对于“*”现象,当出现“*”现象时,通过协议法排除非必要条件ABCDFG,然后观察当“*”现象不存在时发生时,通过差分法排除不充分条件ABCDFG,最终得到E作为充分必要条件。
注意事项:
1.样本量越大,结论越可靠;
2.正例和负例应该足够相似以增强可靠性。
(4)残差法
(A, B, C) 导致 (a, b, c)。
A 导致 a。
B 导致 b。
因此,C 导致 c。
残差法就是在一组因果条件和现象中剔除那些因果关系明确已知的成分,然后得到其余部分构成因果关系。
注意事项:
1.确定AB确实是ab的原因,AB不是c的原因;
2.原因C是否可能是复合原因。
(5)协变法
(A,B,C)和(a,b,c)同时;
(A, B, +C) 和(a, b, +c) 同时;
(A,B,-C)同时发生,(a,b,-c)同时发生;
所以,C 和 c 是因果相关的。
或
(A,B,C)和(a,b,c)同时发生;
(A, B, +C) 和 (a, b, -c) 同时发生;
(A, B, – C) 和 (a, b, +c) 同时发生。
所以,C和c有因果关系。
这其实可以理解为一种数学表达的函数关系,在某些其他变量的情况下,C和c这两个变量域(集)中的元素之间存在一定的对应关系,或者可以正相关,或者可以是负相关的。
注意:
1.C和c之间是否存在唯一的对应关系;
2.C和c之间的函数关系是否可逆。
(6)米尔5法评价
米尔的归纳逻辑思想具有以下三个基本特征:
1.The foundation of the theory of inductive logic is the concept of physical causes. Phenomenons always follow one after another, and they do not happen by chance. Among all the truths related to phenomena, the truth related to the order of the phenomena’ succession is the most important. This is the law of causality. The law of causality is a priori presupposition;
2.inductive science is also the science of argumentation. Their evidence is empirical evidence, and the process is observation and experiment to explore the causal connection between phenomena.
3.Inductive logic should be a method of acquiring new knowledge.
Historical role: Cohen and Nagel have pointed out that any exploration of a phenomenon always begins with a hypothesis In the beginning, without a clear acceptable hypothesis, there can be no experiment or reasoning; at the same time, the hypothesis explaining a phenomenon is often multiple, and the multiple hypothesis needs to be experimentally selected. The function of all experimental criteria is to exclude certain or All selectable factors determine possible causes and effects or constant causal relationships to help people test causal association hypotheses.
Limitations:
1.@ > Exaggerating the role of causal connection in exploring the origin of things, only knowing causal connection is not enough to make the origin of everything in the universe clearly presented to us, and the five methods of seeking causality are only a relatively simple method to determine the causal connection between things. Mill exaggerated the role of these methods, thinking that all causal connections of everything in the universe could be determined based on these methods. This view is one-sided.
2.Mill’s causal connections The identification is also incomplete. The causal connection is understood as the constant successive relationship of the successive relationship. There is an absolute meaning here, which is a kind of deterministic thinking. We know today that the same cause may not always be the same under the same conditions. The same result is produced every time, and the result is random and probabilistic. From this, we will enter the modern inductive logic (probabilistic inductive logic).
(四)The establishment of modern inductive logic And development
Classical inductive logicists dominated by Bacon and Mill believe that inductive logic can not only make scientific discoveries, but also scientific tests, and that inductive logic can achieve the purpose of universal inevitability of knowledge. But this beautiful illusion was completely shattered by Hume’s question.
Hume thought: “In inductive reasoning, there are two logical jumps: one is jumping from a finite instance of actual observation to a jump involving a potential infinity The universal conclusion of the object; the second is a jump from the past and present experience to the prediction of the future. Neither of these are guaranteed by deductive logic, because what applies to the finite does not necessarily apply to the infinite, and the future may be different from the past and the present” .
Based on Hume’s doubts, people gradually changed the cognition that induction can obtain scientific discoveries, and limited the function of induction to evidence. Even within the scope of inspection. In this context, the defense of the “problem of induction” led to the transition from classical inductive logic to modern inductive logic.
With the development of classical probability theory, people gradually realize that introducing probability theory into inductive logic can support inductive logic. De Morgan believes that inductive logic should use probability theory as a tool, and the task of inductive reasoning is to examine the “belief degree” of evidence on the conclusion. The characteristic of inductive reasoning is probability, the conclusion cannot be a universal proposition, but can only be a probability proposition, therefore, inductive logic must be based on probability theory, depart from probability theory, and defend everything done by induction and inductive reasoning are useless.
The establishment of the modern inductive logic theory was marked by the publication of the book “Probability Theory” by the famous British economist Keynes (yes, the buddy of Keynesianism who was criticized by many people) in 1921. Keynes combined probability theory with inductive logic to establish the first probability logic system in the history of world logic. Under the influence of Keynes, logicians have used probability theory as a tool to establish their own inductive logic systems, and the modern inductive logic theory has emerged.
(1)Three schools of modern probability logic
There are quite a few schools of modern inductive logic. The most important schools include the frequentist theory of probability induction, Theories of logicism and privateism.
Logicism understands probability in the sense that probability is a logical relationship between a set of propositions as premises and propositions as conclusions, as Keynes, Carnap, Hinds Deka et al. are represented. The assignment of the probability of this faction is a priori assignment, for example, the probability of drawing a spade from a complete deck of playing cards is 1/4. This probability assignment is based on two assumptions, one is that all The possible situations have been known, and the second is that all possible situations are likely to happen. But we need a relative frequency theory of probability when we cannot predict all possible situations or when these situations are not possible to happen.
Frequentism understands probability in this way, that is, probability refers to the limit of the relative frequency of a certain property or an event in an infinite sequence of time. Represented by Reichenbach and Salmon. This school The probability assignment of is an empirical assignment. For example, the probability of a 50-year-old woman living to 55 years old is 0.97. Such probability calculation is generally performed by taking samples for statistics, such as taking 1000 50-year-old women to form a sample. , after 5 years, if 970 people are still alive, then we can get such a probability. Of course, the sample is always a sample and can only represent local attributes, but if the number of samples reaches a certain number, the probability of the attribute under investigation will remain In order to always show no obvious deviation with the increase of the number, we can temporarily assume that the relative frequency limit has been reached, which can be considered to be close to the real situation. However, whether it is a priori probability assignment or an empirical probability assignment, it is only for a certain For a single event, we need to introduce the theory of privateism.
Privacy understands probability in this way, that is, probability is the actual confidence level of a private person, which can be determined by betting. This confidence is measured. The probability assignment of this faction is a subjective assignment, mainly based on personal preferences and experience or inferences including one’s own knowledge and information in the relevant field. For example, a person thinks that today the Lakers are more than the Pacers. The odds are 8:5, which means he thinks the Lakers have an 8/13 chance of winning.
(2)Statistical Induction
Based on probability theory Let’s talk about statistics. Many people may not be very interested in statistics, and think that this thing is just a statistical report and a record of information. If so, then we may not have the knowledge of statistics. It has attracted enough attention. When we study mathematics in the university, there is a course called “Probability Theory and Mathematical Statistics”. This course is closely related to today’s core preface industrial big data. I will give some examples to let you experience some The role of statistics:
The use of statistics
1.In all kinds of exams from childhood to adulthood, the exam papers you get in your hands are actually statistical questionnaires. By sampling knowledge points, the teacher can master your mastery of the entire knowledge system. In other words, you only need a partial answer to get a score, can be considered to know the knowledge of the entire field of knowledge.
2.The “Father of Epidemiology” surgeon John Snow made this form for cholera:
p>
According to this table, John Snow gave advice: “Stop using Water Company A’s water!” At the time, Snow’s claim was dismissed because of “lack of scientific basis” or “insufficient evidence” Adopted by societies and governments, but following his advice to abandon the use of cholera-contaminated water, towns succeeded in preventing the further spread of cholera.
3.The most important means of modern medicine is called EBM, which translates as “evidence-based medicine”, also called “evidence-based medicine”. The most important part of the above evidence is the statistical data and analysis results obtained through scientific methods.
4.In the presidential campaign against Hillary Clinton, the Trump team used a big data analysis company, Cambridge Analytica, to help the Trump team accurately locate the preferences of American voters and subsequently Advertising, although we dare not assert that Trump’s victory is entirely due to big data, but we can feel the importance of big data in such an important competition, and the foundation of big data is statistics, Trump The general team paid only $15 million in compensation.
Basic Framework of Statistics
To be honest, in the whole course, what I want to talk to you most about is this section. I have been learning and working continuously over the years. In the middle, I have become more and more aware of how important it is to build statistical thinking. Statistics is the science of collecting, arranging, analyzing receipts, and making inferences from data. And if I can give you advice on what is called statistical thinking, what I think of is the following six words “please speak with data”.
I also don’t want to introduce some basic concepts. Let’s start directly from the first principles and focus on the keyword “data”. First of all, we need to start from the source of thinking. We are in daily work. What do you rely more on in debates on various topics in life and even on the Internet? We rely on personal “take for granted”. What is taken for granted? The most basic assumption is our individual feelings and emotions. The intermediate assumption can be obtained by observation and experience. Of course, we can also add some thoughts about our experience. Advanced It is taken for granted that on the basis of mastering knowledge, reasoning and judging problems through knowledge principles. But we know that the world is very complex, the knowledge we already know does not need to be repeated, and the new problems we need to face have no known solutions, so what should we do? The answer I gave was, please let the data speak.
According to the logic knowledge we have learned earlier, we can know that excluding our daily chat is actually expressing a statement. The essence of a statement can be mathematically understood as a functional relationship, that is, Trying to express the mapping relationship between two concept sets or variables, but we know that the world will not directly list the functional relationship to us, it just exists and happens constantly there, and if we intend to find intricate relationships , the functional structure of the two can be obtained at the qualitative or even quantitative level by finding the changes in the data of the two.
A few years ago, there was a best-selling book series called “Freak Economics”. Many students who have a little knowledge of economics may have some doubts, that is, the author Levitt is not describing any economics at all. Knowledge, he is studying some things that are completely irrelevant to the field of economics and production, and is known as the most brain-burning economics book in history, because he has absolutely no idea what he is trying to say. Examples include cheating by teachers and Japanese sumo wrestlers, real estate agents defrauding clients to drive down housing prices, obstetricians increasing the rate of caesarean sections, the strong correlation between crime rates in the US in the 1990s and the legalization of abortion in the 1970s, parenting It has little effect on the child’s success and so on.
Here I will briefly talk about Witt’s analysis of the argument that the decline in crime rates in the 1990s in the United States was mainly due to the legalization of abortion in the 1970s. At first glance, these two things are irrelevant. To prove that it is not possible to do it with our speculative and logical reasoning, even if you can do it, it is not convincing. Levitt analyzed through a lot of research. , get the following data:
1.Before “Roe v. Wade”, five states in the United States had taken the lead in legalizing abortion. The crime rate in these 5 states decreased significantly earlier than other states, and the crime rate decreased more than other states;
2.After the legalization of abortion across the United States, the The actual abortion rate is different. The data show that states with higher abortion rates in the 1970s experienced a greater decline in crime rates in the 1990s; There has been a sharp drop in offenders in young offenders, while offenders in other age groups have not decreased significantly.
Based on the above 3 points, it is eloquently proved that the legalization of abortion was the main reason for the decline of crime rate in the United States in the 1990s.
Such an assertion will naturally arouse various objections on morality and ethics in the American society, so in the face of the question of “whether we should support the legalization of abortion”, according to traditional thinking logic, the positive will It is said that women’s right to free choice is above everything else, while the opposite side believes that a fetus in the womb is the same as a fetus that has already been born, and both are living beings. It’s just a ranking of values, so if someone like Levitt was to look at this issue, what would he think? What economists like to do most is to deal with this kind of moral dilemma, and they will convert this matter into numbers for calculation.
For example, we can assume that the value ratio of infants to fetuses is 1:100 (don’t discuss why this value is here, we are discussing this way of thinking), according to statistics, there are about 160 per year in the United States. More than 10,000 abortions can be converted into 16,000 people killed each year by the Abortion Act. According to Levitt’s data analysis, 16,000 lives are exactly equal to the number of homicides in the United States each year, which is much higher than that due to abortion each year. Legalization reduces the number of homicides. Based on such a comparison of numbers, it becomes clear how to weigh the two, rather than sit there and have endless meetings about the supremacy of life or the supremacy of women’s freedom of choice. proposition.
Speaking of this, what does Levitt’s so-called “devil-like way of thinking” mean? It is to cast aside your value likes and dislikes, blind optimism or vitriolic doubts, cold blood like the devil, and look at the world with cold data rather than inspiration or fantasy.
This is data analysis, which is our statistical thinking. You may ask, isn’t it Freak Economics, what does it have to do with statistics you’re talking about? In fact, I can make such an assertion a little boldly. The mathematical tool of statistics, with the blessing of today’s big data, is probably the most powerful knowledge. As the crown of social sciences – economics, it is the first to put Statistical tools are poured into the discipline, and rigorous mathematical analysis methods are used to study social problems. This is worthy of imitation by other social sciences. In other words, stop using your stereotypes to construct stories and then self Explanation, please go out the door, collect a lot of vast information in the world, and use solid data to prove your point of view, this is the real scientific thinking.
Then speaking of scientific thinking, let me post a chart to show you the scope of application of statistics:
We said earlier It is in the field of social science. According to the method of collecting data, data is divided into observational data and experimental data. Due to the particularity of human society, we are limited by boundaries such as morality and ethics, and it is impossible to conduct some control experiments. Collect observational data for empirical research, but in the field of natural science, we can widely collect data in experiments by controlling experimental subjects. Statistical methods used – large randomised controlled double-blind experiment.
Large sample: It is very simple. The purpose of our statistics is to infer the overall parameters by sampling the population. The larger the sample size, the closer it is to the real population data.
Random: The method of randomization is to give each sampled individual the same probability of being selected from the population. The advantage of this is to eliminate the attribute differences between the sampled individuals;
>
Contrast: When we take random samples, it can be considered that the attribute differences of all samples have been disrupted and eliminated, then our statistics are to find a strong correlation between the variables behind the complex world. The purpose of the comparison is to Changes in control variables;
Double-blind: Double-blind is to keep the person interacting with the subject blinded to the details of the experiment, thereby eliminating the interference of the interactor with the experimental data.
For example, when we make a new drug A, we want to find out its effect on a certain disease B. Then we can conduct random sampling with a large sample among many patients with disease B. The problem to be noted here is that randomness is not random, and randomness is likely to form biased sampling. The essence of randomness is that all individuals have the ability to The same possibility of being drawn, so how do we achieve true randomness? We can assign numbers to all individuals, and extract them through a simple random function to obtain random sampling, so that we can extract patients of various ages, skin colors, family conditions, occupations, etc., which can offset the differences in attributes. .
Next, we set up the control group, that is, the group of patients who did not take the new drug A, and then set the experimental group, that is, the group of patients who took the new drug A (if quantitative research is required, it may be necessary to set up different drug-taking groups). dose), based on double-blind logic, since the control group will know that they have not taken the medicine, we can set up a “placebo group” (logically similar to the control group, but the psychological interference factors of the patients must be excluded), and the whole experimental process, It is operated by a third party without the knowledge of the doctor. Excluding the psychological role of the doctor will affect the objectivity of the experiment and ensure the validity of the experiment.
To sum up, the large-sample randomized controlled double-blind experiment is the “gold standard” used in statistics to test empirical science, and it is not the kind of Western medicine that Chinese medicine understands. The standard of making jokes is not the so-called self-setting standard and self-achieving standard. In other words, whenever a strong correlation needs to be found through practical experiments, this “gold standard” is here. If TCM wants to prove that it is not the kind of utopian metaphysics that is divorced from reality, but a knowledge that can truly stand the test of facts , I’m sorry, you have to get by with statistics.
三、Science and Induction
Finally, we will do a reorganization from scratch. On the premise of the general discussion above, to clearly inform the characteristics or relationships of the local elements in the set, then the really important question is, where does the general discussion of the set as a whole come from? From Bacon to Mill, it should be expanded from the enumeration of individuals to the overall discussion. There is a more important link, that is, how to complete the expansion from local experience to the overall discussion. The scientific method is like this :
The first step is to ask questions. Problems often arise from the inability of old theories to explain new observed phenomena. At this time, scientists will usher in a new opportunity for the problem and obtain a new question worth exploring. The second step is to propose a hypothesis. This is a decisive detail that is different from traditional inductivist philosophers such as Bacon and Mill, that is, the hypothesis is not derived from various empirical data, but a “model” attached to the empirical data by scientists, which is It is the product of thought, and more comes from the transcendence of some kind of genius. It is a purely story structure; the third step, after the hypothesis is constructed, it is accurately and quantitatively described by mathematical tools, and the Use the deductive rigor of mathematics to carry out a series of derivations to obtain various theorems and inferences; the fourth step, under the guidance of the hypothesis, collect and organize data as unbiased as possible to strengthen the probability of the hypothesis or conversely correct the hypothesis .
The above four steps are used continuously and repeatedly, and the discipline system in a certain field will gradually form a system, and future generations can continue to carry out research on the basis.
In today’s era of big data, due to the huge storage and computing functions of computers and the huge underlying logic of statistics, machines can collect massive data through infinite time and space, and perform operations beyond human imagination. The computing speed of the big data matrix is capable of deep self-learning, and the traditional scientific paradigm that requires humans to construct simplified models is likely to be subverted, that is, machines can directly grasp the complex relationships of nodes in the complex world network, which may be worthwhile. a topic to think about.
All in all, inductive logic has developed into statistics and even big data analysis. As a modern human being, it is still the same as the previous sentence. It is necessary for us to keep up with the times and learn to use a mathematical statistical thinking framework to use Speaking of solid data, although this is a more difficult thing (after all, it is more difficult than sitting there thinking about ideas or concepts), but it is still worth doing.
In this way, this course is also a bit of a slogan, and it is all about it. Inductive logic can be regarded as an explanation. In my next session, I will try to learn analogy and metaphorical thinking with you from the field of cognitive science, and I hope you will not dislike it.
Above.
Li Jun, Thursday, April 7, 2022 in Zhanjiang
请登录后发表评论
注册
社交帐号登录